Word clouds and word frequency analysis in qualitative data
In the latest update for Quirkos, we have added a new and much requested feature, word clouds! I'm sure you've used these pretty tools before, they show a random display of all the words in a source of text
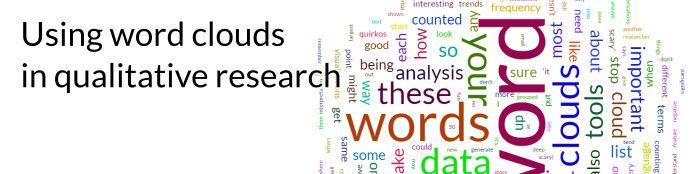
What’s this blog post about? Well, it’s visualised in the graphic above!
In the latest update for Quirkos, we have added a new and much requested feature, word clouds! I'm sure you've used these pretty tools before, they show a random display of all the words in a source of text, where the size of each word is proportional to the number of times it has been counted in the text. There are several free online tools that will generate word clouds for you, Wordle.net being one of the first and most popular.
These visualisations are fun, and can be a quick way to give an overview of what your respondents are talking about. They also can reveal some surprises in the data that prompt further investigation. However, there are also some limitations to tools based on word frequency analysis, and these tend to be the reason that you rarely see word clouds used in academic papers. They are a nice start, but no replacement for good, deep qualitative analysis!
We've put together some tips for making sure your word clouds present meaningful information, and also some cautions about how they work and their limitations.
1. Tweak your stop list!
As these tools count every word in the data, results would normally be dominated by basic words that occur most often, 'the', 'of, 'and' and similar small and usually meaningless words. To make sure that this doesn't swamp the data, most tools will have a list of 'stop' words which should be ignored when displaying the word cloud. That way, more interesting words should be the largest. However, there is always a great deal of variation in what these common words are. They differ greatly between verbal and written language for example (just think how often people might say 'like' or 'um' in speech but not in a typed answer). Each language will also need a corresponding stop list!
So Quirkos (and many other tools) offer ways to add or remove words from the stop list when you generate a word cloud. By default, Quirkos takes the most 50 frequent words from the verbal and written British National Corpus of words, but 50 is actually a very small stop list. You will still get very common words like 'think' and 'she' which might be useful to certain projects looking at expressions of opinions or depictions of gender. So it's a good idea to look at the word cloud, and remove words that aren't important to you by adding them to the stop list. Just make sure you record what has been removed for writing up, and what your justification was for excluding it!
2. There is no weighting or significance
Since word frequency tools just count the occurrence of each word (one point for each utterance) they really only show one thing: how often a word was said. This sounds obvious, but it doesn't give any indication of how important the use of a word was for each event. So if one person says 'it was a little scary', another says 'it was horrifyingly scary' and another 'it was not scary' the corresponding word count doesn't have any context or weight. So this can look deceptive in something like a word cloud, where the above examples count the negative (not scary) and the minor (little scary) the same way, and 'scary' could look like a significant trend. So remember to always go back and read the data carefully to understand why specific words are being used.
3. Derivations don't get counted together
Remember that most word cloud tools are not even really counting words, only combinations of letters. So 'fish', 'fishy' and 'fishes' will all get counted as separate words (as will any typos or mis-spellings). This might not sound important, but if you are trying to draw conclusions just from a word cloud, you could miss the importance of fish to your participants, because the different derivations weren't put together. Yet, sometimes these distinctions in vocabulary are important – obviously 'fishy' can have a negative connotation in terms of something feeling off, or smelling bad – and you don't want to put this in the same category as things that swim. So a researcher is still needed to craft these visualisations, and make decisions about what should be shown and grouped. Speaking of which...
4. They won't amalgamate different terms used by participants
It's fascinating how different people have their own terms and language to describe the same thing, and illuminating this can bring colour to qualitative data or show important subtle differences that are important for IPA[[]] or discourse analysis. But when doing any kind of word count analysis, this richness is a problem – as the words are counted separately. Thus neither term 'shiny', 'bright' or 'blinding' may show up often, but if grouped together they could show a significant theme. Whether you want to treat certain synonyms in the same way is up to the researcher, but in a word cloud these distinctions can be masked.
Also, don’t forget that unless told otherwise (or sometimes hyphenated), word clouds won’t pick up multiple word phrases like ‘word cloud’ and ‘hot topic’.
5. Don’t focus on just the large trends
Word clouds tend to make the big language trends very obvious, but this is usually only part of the story. Just as important are words that aren’t there – things you thought would come up, topics people might be hesitant to speak about. A series of word clouds can be a good way to show changes in popular themes over time, like what terms are being used in political speeches or in newspaper headlines. In these cases words dropping out of use are probably just as interesting as the new trends.

6. This isn't qualitative analysis
At best, this is quantification of qualitative data, presenting only counting. Since word frequency tools are just count sequences of letters, not even words and their meanings, they are a basic supplemental numerical tool to deep qualitative interpretation (McNaught and Lam 2010). And as with all statistical tools, they are easy to misapply and poorly interpret. You need to know what is being counted, what is being missed (see above), and before drawing any conclusions, make sure you understand the underlying data and how it was collected. However…
7. Word clouds work best as summaries or discussion pieces
If you need to get across what’s coming out of your research quickly, showing the lexicon of your data in word clouds can be a fun starting point. When they show a clear and surprising trend, the ubiquity and familiarity most audiences have with word clouds make these visualisations engaging and insightful. They should also start triggering questions – why does this phrase appear more? These can be good points to start guiding your audience through the story of your data, and creating interesting discussions.
As a final point, word clouds often have a level of authority that you need to be careful about. As the counting of words is seen as non-interpretive and non-subjective, some people may feel they ‘trust’ what is shown by them more than the verbose interpretation of the full rich data. Hopefully with the guidance above, you can persuade your audience that while colourful, word clouds are only a one-dimensional dive into the data. Knowing your data and reading the nuance will be what separates your analysis from a one click feature into a well communicated ‘aha’ moment for your field.
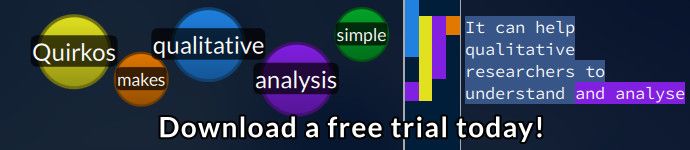
If you'd like to play with word clouds, why not download a free trial of Quirkos? It also has raw word frequency data, and an easy to use interface to manage, code and explore your qualitative data.