Triangulation in qualitative research
Qualitative methods are sometimes criticised as being subjective, based on single, unreliable sources of data. But with the exception of some case study research, most qualitative research will be designed to integrate insights from a variety of data sources
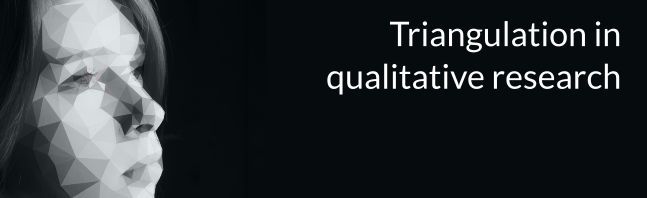
Triangles are my favourite shape,
Three points where two lines meet
- alt-J
What does triangulation mean in qualitative research?
Qualitative methods are sometimes criticised as being subjective, based on single, unreliable sources of data. But with the exception of some case study research, most qualitative research will be designed to integrate insights from a variety of data sources, methods and interpretations to build a deep picture. Triangulation is the term used to describe this comparison and meshing of different data, be it combining quantitative with qualitative, or ‘qual on qual’.
I don’t think of a data in qualitative research as being a static and definite thing. It’s not like a point of data on a plot of graph: qualitative data has more depth and context than that. In triangulation, we think of two points of data that move towards an intersection. In fact, if you are trying to visualise triangulation, consider instead two vectors – directions suggested by two sources of data, that may converge at some point, creating a triangle. This point of intersection is where the researcher has seen a connection between the inference of the world implied by two different sources of data. However, there may be angles that run parallel, or divergent directions that will never cross: not all data will agree and connect, and it’s important to note this too.
What can you triangulate in your research?
You can triangulate almost all the constituent parts of the research process:
- Method triangulation
- Theory triangulation
- Data triangulation
- Investigator triangulation
Data triangulation and method triangulation
What is data triangulation?
Data triangulation, (also called participant or source triangulation) is probably the most common, where you try to examine data from different respondents but collected using the same method. If we consider that each participant has a unique and valid world view, the researcher’s job is often to try and look for a pattern or contradictions beyond the individual experience. You might also consider the need to triangulate between data collected at different times, to show changes in lived experience.
What kinds of qualitative data and methods can be triangulated?
Since every method has weaknesses or bias, it is common for qualitative research projects to collect data in a variety of different ways to build up a better picture. Thus a project can collect data from the same or different participants using different methods, and use method or between-method triangulation to integrate them. Some qualitative techniques can be very complementary, for example semi-structured interviews can be combined with participant diaries or focus groups, to provide different levels of detail and voice. For example, what people share in a group discussion may be less private than what they would reveal in a one-to-one interview, but in a group dynamic people can be reminded of issues they might forget to talk about otherwise.
Method triangulation in mixed-method studies
Researchers can also design a mixed-method qualitative and quantitative study where very different methods are triangulated. This may take the form of a quantitative survey, where people rank an experience or service, combined with a qualitative focus group, interview or even open-ended comments. It’s also common to see a validated measure from psychology used to give a metric to something like pain, anxiety or depression, and then combine this with detailed data from a qualitative interview with that person.
Theoretical triangulation
In ‘theoretical triangulation’, a variety of different theories are used to interpret the data, such as discourse, narrative and context analysis, and these different ways of dissecting and illuminating the data are compared.
Investigator triangulation
Finally there is ‘investigator triangulation’, where different researchers each conduct separate analysis of the data, and their different interpretations are reconciled or compared. In participatory analysis it’s also possible to have a kind of respondent triangulation, where a researcher is trying to compare their own interpretations of data with that of their respondents.
How to do triangulation in qualitative research
While there is a lot written about the theory of triangulation, there is not as much about actually doing it (Jick 1979). In practice, researchers often find it very difficult to DO the triangulation: different data sources tend to be difficult to mesh together, and will have very different discourses and interpretations. If you are seeing ‘anger’ and ‘dissatisfaction’ in interviews with a mental health service, it will be difficult to triangulate such emotions with the formal language of a policy document on service delivery.
In general the qualitative literature cautions against seeing triangulation as a way to improve the validity and reliability of research, since this tends to imply a rather positivist agenda in which there is an absolute truth which triangulation gets us closer to. However, there are plenty that suggest that the quality of qualitative research can be improved in this way, such as Golafshani (2003). So you need to be clear of your own theoretical underpinning: can you get to an ‘absolute’ or ‘relative’ truth through your own interpretations of two types of data? Perhaps rather than positivist this is a pluralist approach, creating multiplicities of understandings while still allowing for comparison.
It’s worth bearing in mind that triangulation and multiple methods isn’t an easy way to make better research. You still need to do all different sources justice: make sure data from each method is being fully analysed, and iteratively coded (if appropriate). You should also keep going back and forth, analysing data from alternate methods in a loop to make sure they are well integrated and considered.
Software for qualitative triangulation
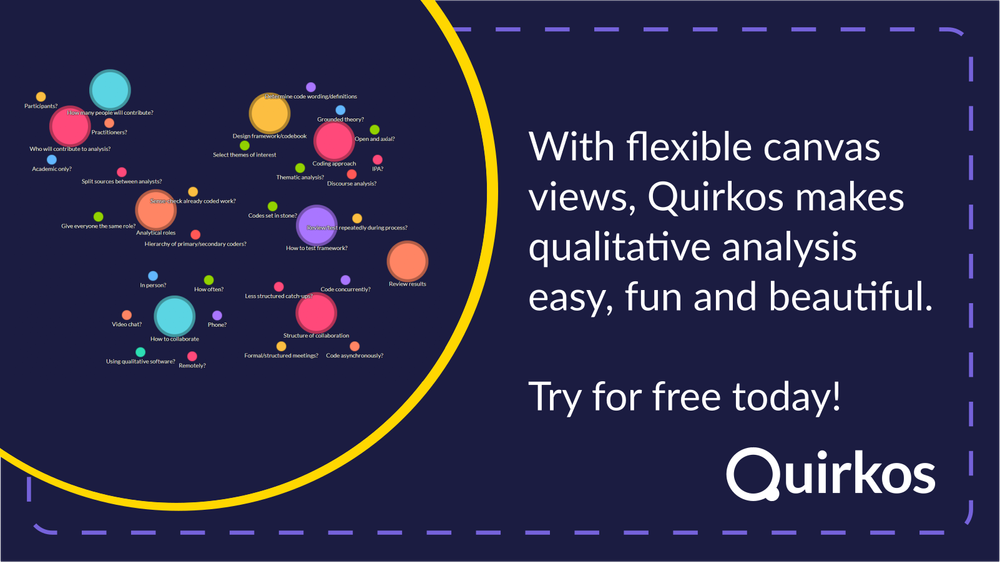
Qualitative data analysis software can help with all this, since you will have a lot of data to process in different and complementary ways. In software like Quirkos you can create levels, groups and clusters to keep different analysis stages together, and have quick ways to do sub-set analysis on data from just one method. Check out the features overview or mixed-method analysis with Quirkos for more information about how qualitative research software can help manage triangulation.
References and further reading
Carter et al. 2014, The use of triangulation in qualitative research, Oncology Nursing Forum, 41(5), https://www.ncbi.nlm.nih.gov/pubmed/25158659
Denzin, 1978 The Research Act: A Theoretical Introduction to Sociological Methods, McGraw-Hill, New York.
Golafshani, N., 2003, Understanding reliability and validity in qualitative research, 8(4), https://nsuworks.nova.edu/cgi/viewcontent.cgi?article=1870&context=tqr
Bekhet A, Zauszniewski J, 2012, Methodological triangulation: an approach to
understanding data, Nurse Researcher, 20 (2), https://journals.rcni.com/doi/pdfplus/10.7748/nr2012.11.20.2.40.c9442
Jick, 1979, Mixing Qualitative and Quantitative Methods: Triangulation in Action, Administrative Science Quarterly, 24(4), https://www.jstor.org/stable/2392366