Quantitative vs. qualitative research
So this much is obvious: quantitative research uses numbers and statistics to draw conclusions about large populations. You count something that is countable, and process results across the sample. Qualitative methods are more elusive
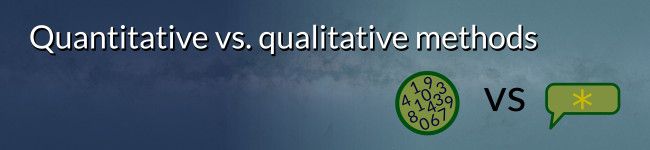
So this much is obvious: quantitative research uses numbers and statistics to draw conclusions about large populations. You count something that is countable, and process results across the sample.
Qualitative methods are more elusive: however in general they revolve around collecting data from people about an experience. This could be how they used a service, how they felt about something, and could be verbal or written. But it is generally speech or talk, albeit with a variety of levels inferred above and below this (if they are truthful, or if what they say has deeper or hidden meaning). Rather than applying a statistical test to the data, a qualitative researcher must read/listen to the data and make an interpretation of what is being discussed, often hoping to discover patterns or contradictions.
Interpretation is done in both approaches: quantitative results are still examined in context (often compared with other numbers and data), and given a metric of significance such as a p-value or r-squared to assess if the results, or a part of them, are meaningful.
Finally, in general it is seen that a quantitative approach is a positivist paradigm, while qualitative methods fit better with constructionist or pragmatic paradigms (Savin-Baden and Major 2013). However, both are essentially attempting to model and sample something about the world in which we live so that we can simplify and understand it. And it’s not a case of one is better than the other: just like a hammer can’t be used to turn screws, or a screwdriver to hammer in nails, the different methods have different uses. Researchers should always make sure that the question comes first, and that is used to choose the methodology.
But you should also ask, is there a quantitative way to measure what your question is asking? If it’s something as simple as numbers of people, or a quantitative aspect like salary. While there are also quantitative measures of things like anxiety or pain that can be used as a proxy to make inferences across a large population. However, for detailed understanding of these issues and how they affect people, these metrics can be crude and don’t get to the detail of the lived experience.
However, choosing the right approach also depends on the how much is known about the research question and topic area. If you don’t know what the problems are in a field, you don’t know what questions to ask, or how to record the the answers.
I would argue that even in the physical sciences, qualitative research comes first, and sets questions to answer with quantitative methods. Quantitative research projects usually grow from qualitative observations of the physical world, such as 'I can see that ice seems to melt when it gets warm. At what temperature does ice melt?' or qualitative exploration of the existing literature to find things from other research that is surprising or unexplained.
In the classic high-school science experiment above, you would quantitatively measure the melting point of water by taking a sample. You don't try and melt all the ice in the world: you take one piece, and assume that other ice behaves in the same way. In both quantitative and qualitative research, sampling correctly is important. Just as only taking one small piece of impure ice will give you skewed results, so will only sampling one small part of a human population.
In quantitative research, because you are usually only sampling for one question at a time (i.e. temperature) it's best to have a large sample size. Especially when dealing with naturally variable, unrestricted variables (for example like a person's height) the data will tend to form a bell curve with a large majority of the answers in the middle, and a small number of outliers at either end. If we were sampling ice to melt, we might find that most ice melts around the same temperature, but very pure or dirty ice will have a slight difference. We would take the answer to be the statistical average, for the mean by adding up all the results and dividing by the sample size.
You could argue that the same is true for qualitative research. If you are asking people about their favourite ice cream, you'll get a better answer by asking a large number of people, right? Well this might not always be true. Firstly, just as with the ice melting experiment, sampling every piece of ice in the world will not add much more accuracy to your work but will be a lot more work. And with qualitative research, you are generally asking a much more complicated question for each person sampled, so the work increases exponentially as your sample size grows.
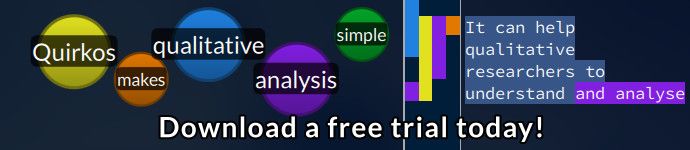
As your qualitative data grows, Quirkos can help you manage and make sense of it...
Remember, it's rare that qualitative research aims to give one definitive answer: it's more exploratory, and interested in the outlier cases just as much as the common ones. So in our qualitative research question 'What is your favourite ice cream' people may talk about gelato, sorbet or iced coffee. Are these really ice cream? One could argue that technically they are not, but if people consider them to be ice cream, and we want to know what to sell for desert at our restaurant, this becomes relevant. As a result of qualitative research, we usually learn to ask better questions 'What is your favourite frozen dessert?' might be a better question.
Now our qualitative research has helped us create a good piece of quantitative research. We can do a survey with a large sample size, and ask the question 'What is your favourite frozen dessert?' and give a list of options which are the most common answers from our qualitative research.
However, there can still be flaws with this approach. When answering a survey people don't always say what they mean, and you lose the context of their answers. In surveys there is primacy effect which means that people are lazy, and much more likely to tick the first answer in a list. In this case, the richness of our qualitative answers are lost. We don't know what context people are talking about (while walking along a beach, or in a restaurant or at home?) and we also loose the direct contact with the respondent so we can tell if they are lying or being sarcastic, and we can't ask follow on questions.
That's why qualitative research can still be useful as part of, or following quantitative research, for discovering ‘Why’ – understanding the results in the richness of lived experience. Often research projects will have a qualitative component – taking a subset of the the larger quantitative study and getting an in-depth qualitative insight with them.
There’s no shame in using a mixed methods approach if it is the most appropriate for the subject area. While there is often criticism over studies that ‘tack-on’ a small qualitative component, and don’t properly integrate or triangulate the types of results, this is a implementation rather than paradigm problem. But remember, it’s not a case of one approach vs another, there are no inheriently good or bad approaches. Methods should be appropriate to each task/question and should be servants to the researcher, not ruling them (Silverman 2013).
Quirkos is about as close as a pure qualitative software package as you can find. It's quick to learn, visual and keeps you close to the data. Our focus is on just doing qualitative coding and analysis well, and not to attempt statistical analysis of qualitative data. We believe that for most qualitative researchers that's the right methodological approach. However, there is capacity for allow some mixed method analysis, so that you can filter results by demographic or other data.
The best way to see if Quirkos works for you is to give it a go! Download our one month free trial of the full version with no restrictions, and see if Quirkos works for your research paradigm.