Co-producing analysis: exploring together
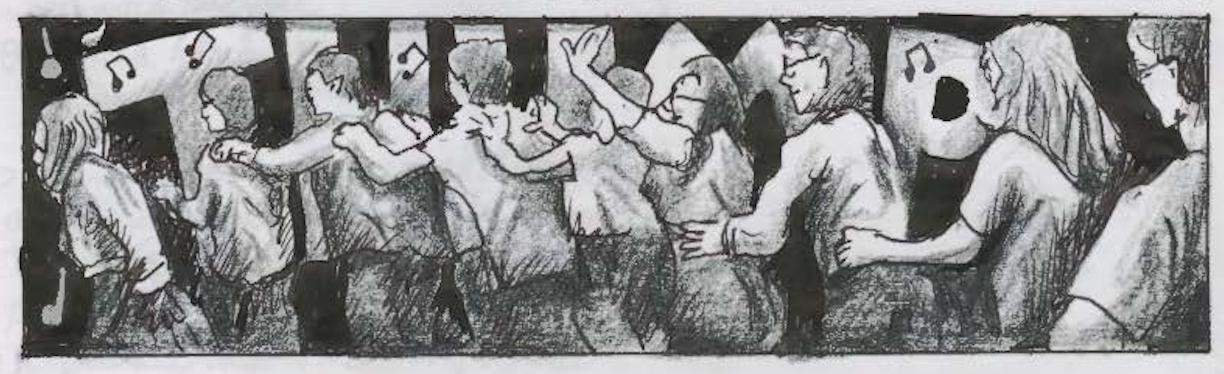
This guest blog-post ties in with the International Creative Research Methods Conference 2024, by Dr Jess Mannion: a Lecturer and Researcher in the Department of Social Care and Social Work at Manchester Metropolitan University. She specialises in co-produced research with people with learning disabilities on sexual citizenship using visual and creative research methods and action research.
Why in co-produced studies are participants generally not engaged in the data analysis stage? Could this involvement result in more robust findings and deeper insights?
During a co-produced project I was working on with a team of co-researchers with learning disabilities (Mannion, 2024), we began to question how we could co-analyse our data together. We wanted to ensure best practices in participatory research where everyone was involved, from design to data generation, to analysis, and sharing the findings. Studies where participants are engaged at this level of collaboration are rare, particularly in learning disability research. This was even more evident when it came to the data analysis stage where there was little guidance in the literature.
Data analysis can be a complex area for inexperienced co-researchers, so participants are usually excluded, despite its importance. Kara (2015) argued that if a study is to be fully inclusive, then the option to be engaged in the data analysis stage should be available. If participants are engaged in analysis, they are not only involved in all stages of research, but it adds a deeper layer to the findings. Together they can check if anything was omitted, misinterpreted, or needs to be taken out, and the meaning on important topics can be explored further.
This research already used visual and creative research methods to elicit the data, but as there was little guidance in the literature on how to analyse the data together in a meaningful and accessible way, we decided to create a new method of data analysis. Drawing from existing ideas and data analysis methods in the literature, we devised a systematic mixed method, named ‘Creative, collaborative data analysis’ that would be accessible and interesting. These ideas came from visual analysis (Kara et al., 2021), member checking (Call-Cummins et al., 2019), content analysis (Grbich, 2013), and embodied data analysis (Thanem and Knights, 2019).
As data analysis progressed, it resulted in the co-creation of the method with the co-researchers who contributed ideas to the development of the method. For example, in step 5 (explored below) we brought all the study’s data together in one space and decided to see what would happen. Here, one co-researcher suggested we each take what was important to us or stood out to explore further. Then in stage 6 (described below) the co-researchers devised how we could reflect at the end of the project by designing a research question and a method to explore it. This co-creation made this data analysis method genuinely collaborative.
The data analysis method is summarised below, but for further insight into this method see (Mannion, 2024b).
Creative collaborative data analysis method.
- Visual analysis:
The participants should choose a topic to research and a visual and creative research method to help them explore it. After creating this, it should be shared along with its meaning for group discussion. This discussion should be recorded and transcribed.
- Member checking:
Member checking can help ensure the interpretations gathered are accurate and give additional opportunities to discuss the findings with the participants as perspectives change over time. The researcher should listen to the recording of each data collection meeting and make a 10-minute video summarising the main interpretations. Before the next meeting, the video should be sent to participants along with reflective questions so they can respond to the interpretation of the findings. Here, they should vocalise if interpretations are incorrect and if data should be added or omitted.
- Content analysis:
The textual data should be systematically categorised by finding patterns in the words used. The repetition of words is presumed to signify importance (Gbrich, 2013). The researcher should identify word frequency by counting and selecting the main words in the transcripts, omitting words such as ‘a’, ‘in’, and ‘the’. The keywords should be written on postcards. This approach leaves more manageable data for the participants to work with but will enable meaningful engagement with the data, where the participants can dig deeper into interpretations and provide a more complete picture.
- Arts based analysis:
The word cards should be placed on a table with participants seated around them. Each participant should be asked to choose a word that is most important to them and create a visual with drawings and words to express its meaning. They should then be asked to share their visuals and interpretations. This should lead to further group discussion, where the main themes are decided upon based on what is most important.
- Embodied data analysis:
All artefacts collected during the study through the creation of the visual and creative research methods to collect the data should be displayed on a large table. This can include items such as theme word cards, artwork, and poetry. Any audio recorded can be played in the background; for instance, we made raps. Participants should be asked to move around and play with the data. They should then be asked to lift artefacts and/or themes from the table that are important or stand out. They can write words on blank postcards if they are choosing the background sound to represent it. Participants should then share what they have chosen and why, which should lead to a group discussion. All other data should be removed, and participants asked to place their data on the table. While discussing the data, the participants should decide what the main themes are, decide what data can be placed together as sub-themes, and remove any data they feel is irrelevant.
A later research meeting should be held where the participants can review their analysis. Each piece of removed data should be presented to participants to ensure they have not missed anything. This will give the opportunity to debate the data further, create further sub-themes, and remove any repeated or irrelevant data from their previous analysis.
- Creative collaborative reflection:
The participants should decide what questions to ask to reflect on the project at its end and what visual and creative research methods they would like to use to help them do this. For example, we used the 'third objects' method, where each participant brought in a found or made object to represent the ‘biggest thing they were taking from the group’. The participants should share their metaphors and interpretations, leading to a group discussion.
For this method to work well, I recommend that the participants have strong working relationships over an extended period. Although the co-researchers found some of the method creation and analysis hard, they found it fun and interesting. It provided a platform for them to be heard, and to gain insight into other people's perceptions. The co-researchers learnt about data analysis and their confidence grew in the process. Our co-creation of a new, creative, collaborative, and accessible method of data analysis is a contribution to research and will provide future opportunities for participants in co-produced studies to analyse their data.
To read more about the Creative Collaborative Data Analysis method, for which I have authored a chapter, and other creative data analysis methods, see ‘The Handbook of Creative Data Analysis’ edited by Helen Kara, Dawn Mannay, and Alastair Roy Policy Press | The Handbook of Creative Data Analysis, Edited by Helen Kara, Dawn Mannay and Alastair Roy (bristoluniversitypress.co.uk). Thank you to the Relationships and Sexuality Research Team who co-created this data analysis method.
References:
Call-Cummings, M., Hauber-Özer, M., Byers, C. and Mancuso, G.P. (2019) The power of/in photovoice, International Journal of Research & Method in Education, 42(4): 399-413.
Grbich, C. (2013) Qualitative Data Analysis: An Introduction. London: Sage Publications.
Kara, H. (2015) Creative research methods in the social sciences. A practical guide. Bristol: Policy Press. Kara, H., Lemon, N., Mannay, D. and McPherson, M. (2021) Creative Research Methods in Education: Principles and Practices. Bristol: Policy Press.
Mannion, J. (2024) Establishing a Cooperative Inquiry Group for People with Intellectual Disabilities to Explore Relationships and Sexuality in their Lives., Trinity College Dublin, School of Nursing & Midwifery, Nursing.
Mannion, J. (2024b)‘Creative collaborative data analysis: Co-constructing and co-analysing the data together’ in, editors Kara, C., Mannay, D. & Alastair, R., Handbook of creative data analysis. Bristol: Policy Press.
Thanem, T. and Knights, D. (2019) Embodied Research Methods, London: SAGE.
Image by Stephen D. Kroeger, Ed.D., Associate Professor Emeritus, School of Education, University of Cincinnati