6 meta-categories for qualitative coding and analysis
When doing analysis and coding in a qualitative research project, it is easy to become completely focused on the thematic framework, and deciding what a section of text is about. However, qualitative analysis software is a useful tool for organising more
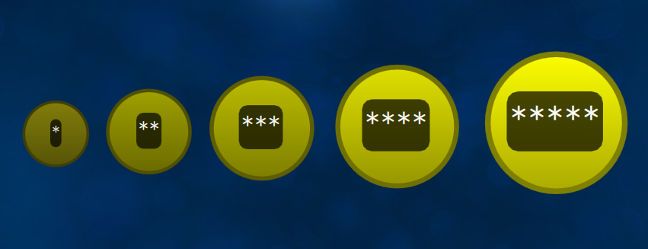
When doing analysis and coding in a qualitative research project, it is easy to become completely focused on the thematic framework, and deciding what a section of text is about. However, qualitative analysis software is a useful tool for organising more than just the topics in the text, they can also be used for deeper contextual and meta-level analysis of the coding and data.
Because you can pretty much record and categorise anything you can think of, and assign multiple codes to one section of text, it often helps to have codes about the analysis that help with managing quotes later, and assisting in deeper conceptual issues. So some coders use some sort of ranking system so they can find the best quotes quickly. Or you can have a category for quotes that challenge your research questions, or seem to contradict other sources or findings. Here are 6 suggestions for these meta-level codes you could create in your qualitative project (be it Quirkos, Nvivo, Atlas-ti or anything!):
Rating
I always have a node I call ‘Key Quotes’ where I keep track of the best verbatim snippets from the text or interview. It’s for the excited feeling you get when someone you interviewed sums up a problem or your research question in exactly the right way, and you know that you are going to end up using that quote in an article. Or even for the title of the article!
However, another way you can manage quotes is to give them a ranking scheme. This was suggested to me by a PhD student at Edinburgh, who gives quotes a ranking from 1-5, with each ‘star-rating’ as a separate code. That way, it’s easy to cross reference, and find all the best quotes on a particular topic. If there aren’t any 5* quotes, you can work down to look at the 4 star, or 3 star quotes. It’s a quick way to find the ‘best’ content, or show who is saying the best stuff. Obviously, you can do this with as little or much detail as you like, ranking from 1-10 or just having ‘Good’ and ‘Bad’ quotes.
Now, this might sound like a laborious process, effectively adding another layer of coding. However, once you are in the habit, it really takes very little extra time and can make writing up a lot quicker (especially with large projects). By using the keyboard shortcuts in Quirkos, it will only take a second more. Just assign the keyboard numbers 1-5 to the appropriate ranking code, and because Quirkos keeps the highlighted section of text active after coding, you can quickly add to multiple categories. Drag and drop onto your themes, and hit a number on the keyboard to rank it. Done!
Contradictions
It is sometimes useful to record in one place the contradictions in the project – this might be within the source, where one person contradicts themselves, or if a statement contradicts something said by another respondent. You could even have a separate code for each type of contradiction. Keeping track of these can not only help you see difficult sections of data you might want to review again, but also show when people are being unsure or even deceptive in their answers on a difficult subject. The overlap view in Quirkos could quickly show you what topics people were contradicting themselves about – maybe a particular event, or difficult subject, and the query views can show you if particular people were contradicting themselves more than others.
Ambiguities
In qualitative interview data where people are talking in an informal way about their stories and lives, people often say things where the meaning isn’t clear – especially to an external party. By collating ambiguous statements, the researcher has the ability to go back at the end of the source and see if each meaning is any clearer, or just flag quotes that might be useful, but might be at risk of being misinterpreted by the coder.
Not-sures
Slightly different from ambiguities: these are occasions when the meaning is clear enough, but the coder is not 100% sure that it belongs in a particular category. This often happens during a grounded theory process where one category might be too vague and needs to be split into multiple codes, or when a code could be about two different things.
Having a not-sure category can really help the speed of the coding process. Rather than worrying about how to define a section of text, and then having sleepless nights about the accuracy of your coding, tag it as ‘Not sure’ and come back to it at the end. You might have a better idea where they all belong after you have coded some more sources, and you’ll have a record of which topics are unclear. If you are not sure about a large number of quotes assigned to the ‘feelings’ Quirk (again, shown by clustering in the overlap view in Quirkos), you might want to consider breaking them out into an ‘emotions’ and ‘opinions’ category later!
Challenges
I know how tempting it can be to go through qualitative analysis as if it were a tick-box exercise, trying to find quotes that back up the research hypothesis. We’ve talked about reflexivity before in this blog, but it is easy to go through large amounts of data and pick out the bits that fit what you believe or are looking for. I think that a good defence against this tendency is to specifically look for quotes that challenge you, your assumptions or the research questions. Having a Quirk or node that logs all of these challenges lets you make sure you are catching them (and not glossing over them) and secondly provides a way to do a validity assessment at the end of coding: Do these quotes suggest your hypothesis is wrong? Can you find a reason that these quotes or individuals don’t fit your theory? Usually these are the most revealing parts of qualitative research.
Absences
Actually, I don’t know a neat way to capture the essence of something that isn’t in the data, but I think it’s an important consideration in the analysis process. With sensitive topics, it is sometimes clear to the researcher that an important issue is being actively avoided, especially if an answer seems to evade the question. These can be at least coded as absences at the interviewer’s question. However, if people are not discussing something that was expected as part of the research question, or was an issue for some people but not others, it is important to record and acknowledge this. Absence of relevant themes is usually best recorded in memos for that source, rather than trying to code non-existent text!
These are just a few suggestions, if you have any other tips you’d like to share, do send them to daniel@quirkos.com or start a discussion in the forum. As always, good luck with your coding!